The Epic Python Developer Certification Bundle
1268 Enrolled
12 Courses & 91 Hours
$39.99$180.00
You save 77%
What's Included
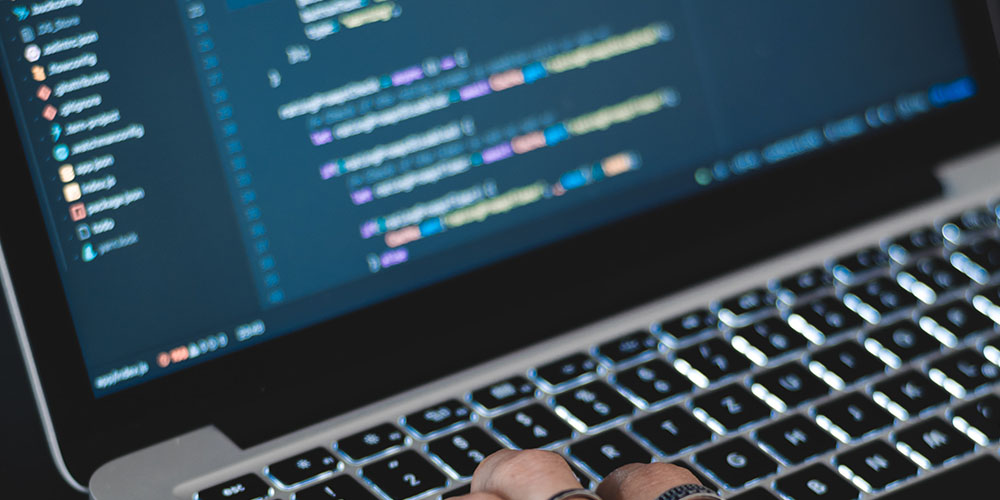
$15.00 Value
Complete Data Science Training with Python for Data Analysis
Minerva Singh
116 Lessons (12h)
Lifetime
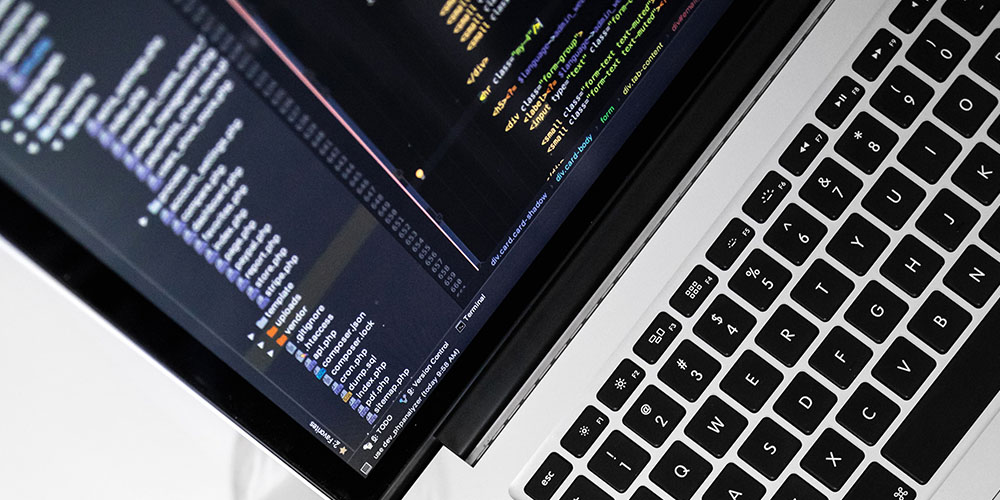
$15.00 Value
Master Clustering Analysis for Data Science Using Python
Nouman Azam
35 Lessons (5h)
Lifetime
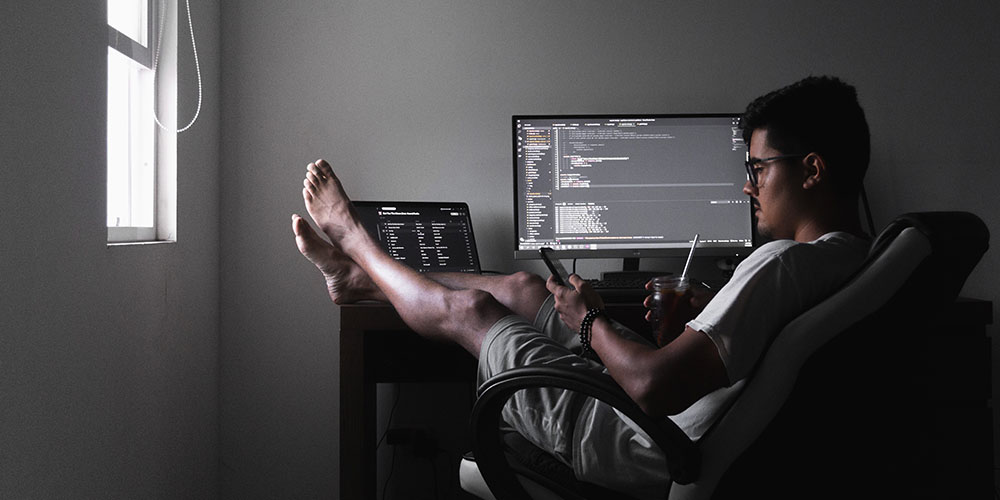
$15.00 Value
Python 3 Complete Masterclass: Make Your Job Tasks Easier!
Mihai Catalin Teodosiu
246 Lessons (15h)
Lifetime
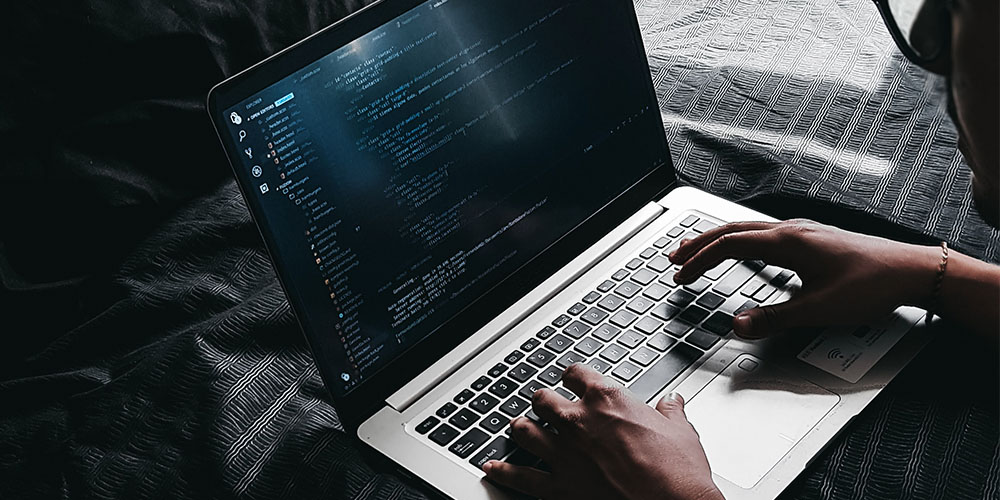
$15.00 Value
Python 3 Network Programming: Build 5 Network Apps
Mihai Catalin Teodosiu
200 Lessons (10h)
Lifetime
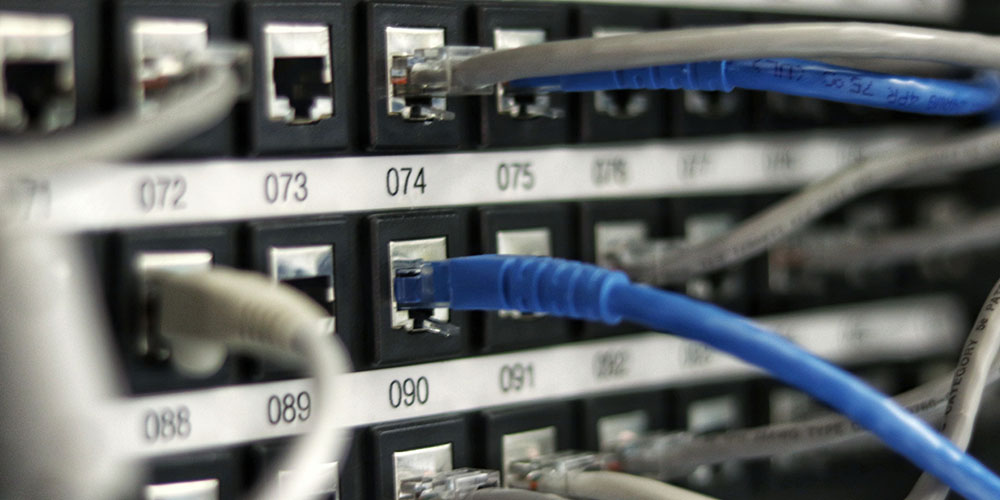
$15.00 Value
Python 3 Network Programming – Sequel: Build 5 More Apps
Mihai Catalin Teodosiu
62 Lessons (3h)
Lifetime
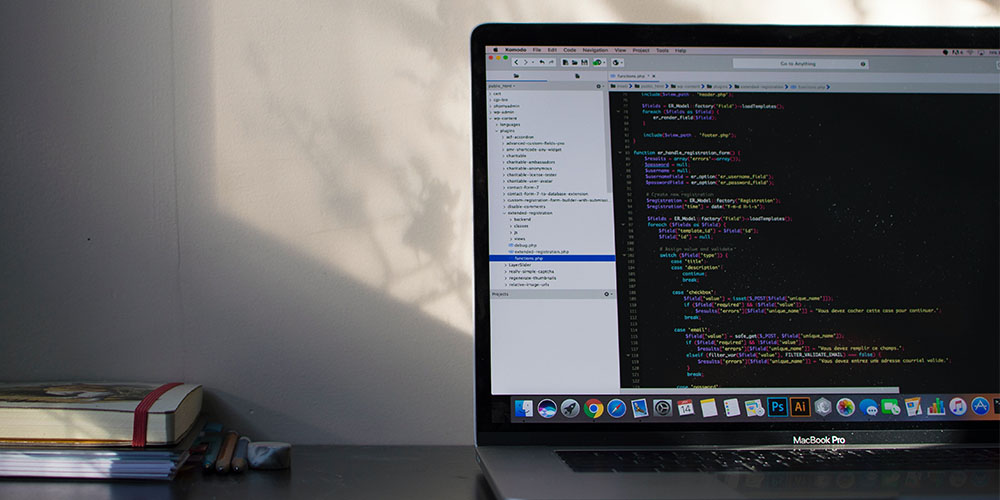
$15.00 Value
Learning Python 3 Programming for the Absolute Beginner
Lee Assam
106 Lessons (11h)
Lifetime
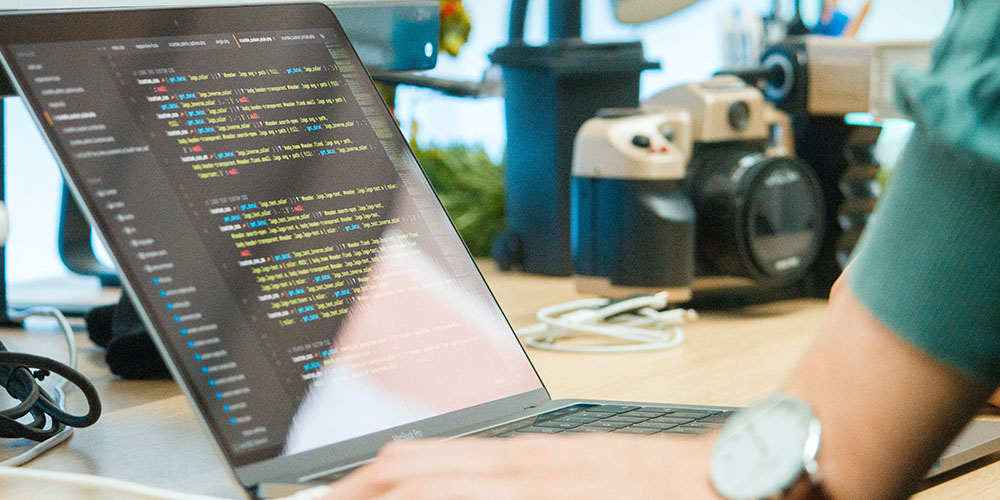
$15.00 Value
Learn Python 3 from Beginner to Advanced
Ermin Kreponic
73 Lessons (8h)
Lifetime
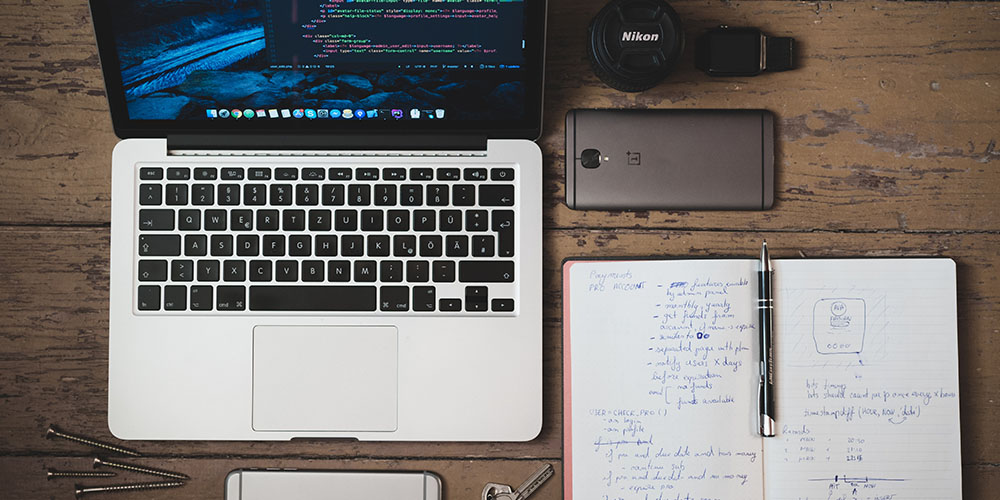
$15.00 Value
Python for Beginners: Learn Python from Scratch
Arkadiusz Wlodarczyk
70 Lessons (10h)
Lifetime
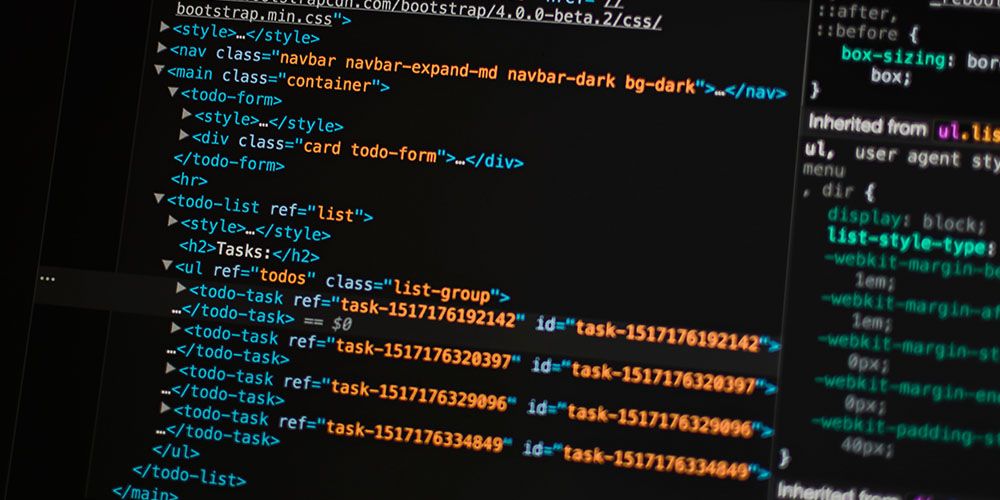
$15.00 Value
Statistics & Machine Learning Techniques for Regression Analysis with Python
Minerva Singh
50 Lessons (6h)
Lifetime
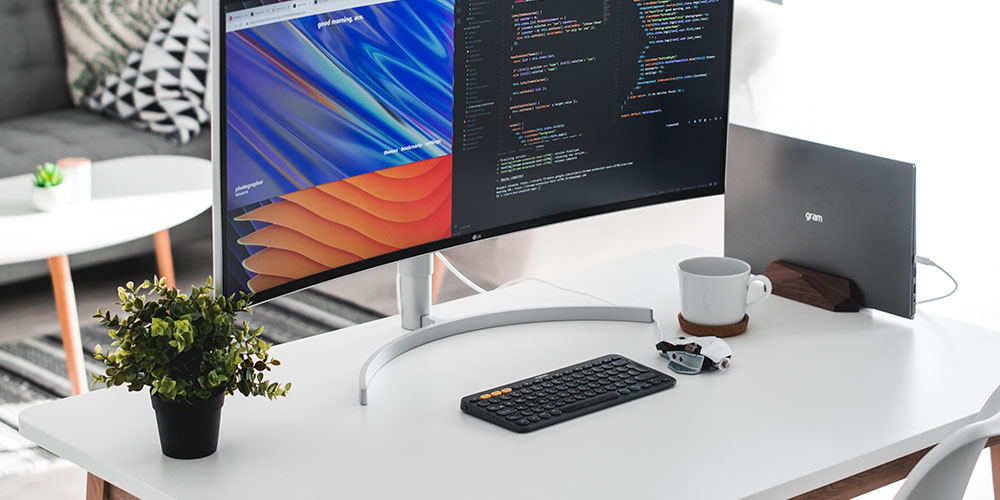
$15.00 Value
Working with Classes: Classify & Cluster Data with Python
Minerva Singh
46 Lessons (4h)
Lifetime
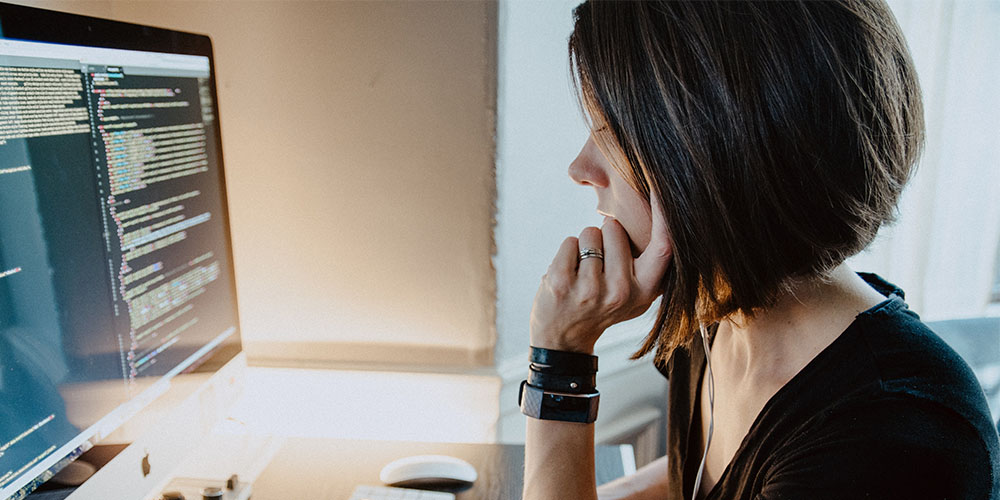
$15.00 Value
Practical Data Pre-Processing & Visualization Training With Python
Minerva Singh
49 Lessons (5h)
Lifetime
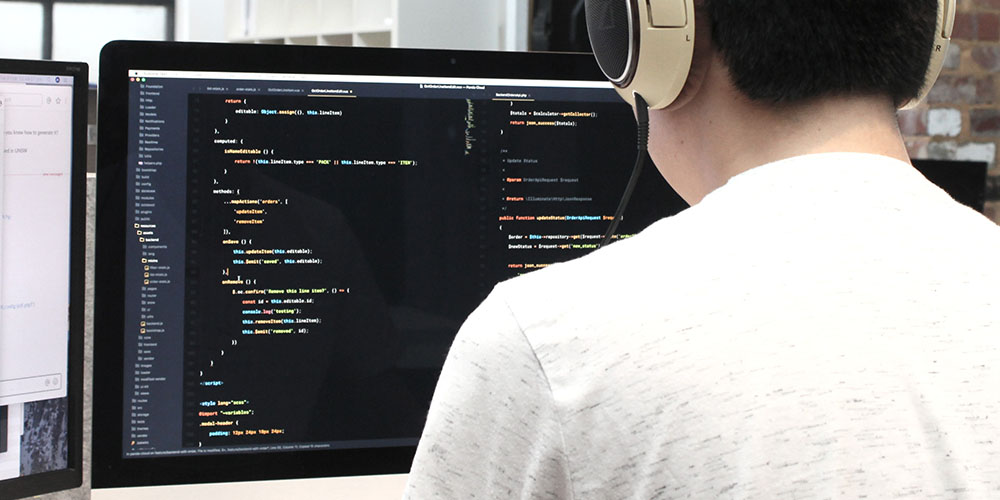
$15.00 Value
Python 3 for Offensive PenTest: A Complete Practical Course
Nouman Azam
28 Lessons (2h)
Lifetime
Terms
- Unredeemed licenses can be returned for store credit within 30 days of purchase. Once your license is redeemed, all sales are final.
4 Reviews
5/ 5
All reviews are from verified purchasers collected after purchase.
CS
CHRISTOS SEITANIDIS
Verified Buyer
Yes, it's epic as a developer bundle for Python! It has so much as variety of content and great exposition!
Jul 20, 2021
DE
Dev Elgert
Verified Buyer
I just started the course but the videos are great and informative.. I will update my review when I am close to completing it.
Mar 4, 2021
BG
Brian Goulter
Verified Buyer
From first impressions, this looks good. There is a lot of free training available on Youtube, but it is nice not having to search for the material that suits my learning style.
Feb 10, 2021
Netaji Kakani
Verified Buyer
I love the breath and depth of this course can’t wait to complete all topics in the course and also instructors have such accomplished backgrounds
Jul 4, 2020
Your Cart
Your cart is empty. Continue Shopping!
Processing order...